pg_search: Elastic-Quality Full Text Search Inside Postgres
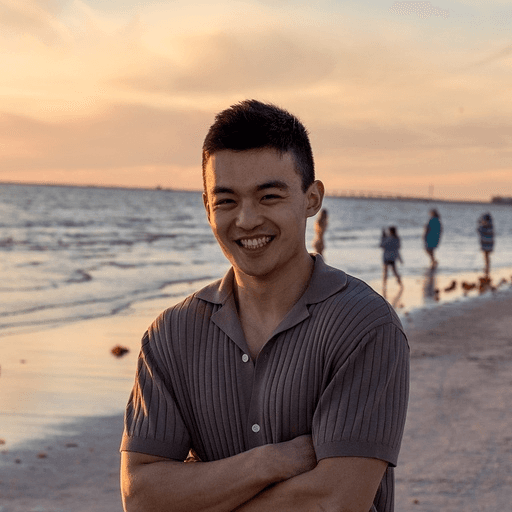
By Ming Ying on October 1, 2023
With the release of v0.6.0
, which marks the first stable release of the
ParadeDB full-text search extension, we have renamed pg_bm25
to pg_search
.
We're unveiling pg_search
: a Rust-based Postgres extension that significantly improves Postgres' full text
search capabilities. pg_search
is named after BM25, the algorithm used by modern search engines to calculate the
relevance scores of search results.
Today, Postgres' native full text search, which uses the tsvector
type, has two main problems:
- Performance: Searching and ranking over large tables is sluggish. When tables grow to millions of rows, a single full text search can take several minutes.
- Functionality: Postgres has no support for operations like fuzzy search, relevance tuning, or BM25 relevance scoring, which are the bread and butter of modern search engines.
pg_search
aims to bridge the gap between the native capabilities of Postgres’ full text search and those of a specialized search engine like Elasticsearch.
The goal is to eliminate the need to bring a cumbersome service like Elasticsearch into the data stack.
Some features of pg_search
include:
- 100% Postgres native, with zero dependencies on an external search engine
- Built on top of Tantivy, a Rust-based alternative to the Apache Lucene search library
- Query times over 1M rows are 20x faster compared to
tsquery
andts_rank
, Postgres' built-in full text search and sort functions - Support for fuzzy search, aggregations, highlighting, and relevance tuning
- Relevance scoring uses BM25, the same algorithm used by Elasticsearch
- Real-time search — new data is immediately searchable without manual reindexing
pg_search
stands on the shoulders of several open-source giants. The goal of this blog post is to recognize these projects
and to share how pg_search
was built.
The Shoulders of Giants
Putting a search engine inside of Postgres is hard. A few projects have attempted it, but with one caveat: every single one has relied on an external Elasticsearch instance. This means introducing a complex and expensive piece of infrastructure into the data stack. Perhaps the best-known example of this kind of design is a Postgres extension called ZomboDB.
In 2016, an open source search library called Tantivy emerged. Tantivy was designed as a Rust-based alternative to Apache Lucene, the search library that powers Elasticsearch. Three years later, a library called pgrx — built by the same author of ZomboDB — made it possible to build Postgres extensions in Rust. Combined, these projects laid the groundwork for a Postgres extension that could create Elastic-quality search experiences within Postgres.
Creating the Inverted Index
Like Elasticsearch, the backbone of Tantivy's search engine is a data structure called the inverted index, which stores a mapping from words to their locations in a set of documents. An inverted index is like the table of contents of a book — without it, you might have to examine every page to find a specific chapter.
Rather than creating this inverted index externally, pg_search
stores the
index inside Postgres as a new, Postgres-native index type, which we call the BM25 index. This is made possible
through Postgres' index access method API.
When a BM25 index is created, Postgres automatically updates it as new data arrives
or is deleted in the underlying SQL table. In this way, pg_search
enables real-time search without any
additional reindexing logic.
Building the SQL Interface
Following index creation, the next step was to expose an intuitive SQL interface for users to write search queries.
This was accomplished through the Postgres operator API,
which enables the creation of custom Postgres operators. We chose the @@@
operator to signify the beginning
of a query to the BM25 index in homage to the @@
operator used by Postgres' native full text search.
The end result is the ability to search any table with a single SQL query.
SELECT *
FROM my_table
WHERE my_table @@@ ('idx_mock_items', '"my query string"')
Wherever possible, we designed the SQL interface, ParadeQL, to transparently mirror Tantivy's API. For instance,
the right-hand side of the @@@
operator accepts Tantivy's mini query language and configuration options.
SELECT *
FROM my_table
WHERE my_table @@@ 'description:keyboard^2 OR electronics:::fuzzy_fields=description&distance=2'
Performance Benchmarks
On a table with one million rows, pg_search
indexes 50 seconds faster than tsvector
and ranks results
20x faster. Indexing and search times are nearly identical to those of a dedicated Elasticsearch instance.
With further optimizations, we're aiming to reduce the query times compared to Elasticsearch
by an additional 2x.
More detailed benchmark results can be found in the README.
Wrapping Up
pg_search
is ready for use today. There are two ways to try it: installing it inside an existing, self-hosted Postgres instance,
or running the Postgres Docker image.
pg_search
is open-source and licensed under AGPL. If you'd like to contribute, the best place to start is our
Slack community. And please don't hesitate to show your support by
giving us a star!